Health Analysis
Solving Everyday Problems Through Applied Research
Importance of Health Analysis
Health analysis is crucial for assessing the overall well-being of individuals and populations, guiding decisions in healthcare, public policy, and preventive measures. By systematically examining health data—such as vital signs, laboratory results, and medical histories—health professionals can identify patterns, diagnose conditions, and monitor disease progression. This analysis plays a central role in detecting risk factors early, enabling timely interventions that prevent chronic illnesses or manage existing conditions more effectively. Additionally, health analysis supports the development of personalized treatment plans, ensuring that medical care is tailored to the unique needs of each patient, which improves outcomes and quality of life.
On a broader scale, health analysis is vital for public health initiatives and healthcare system management. It provides critical insights into population health trends, such as the spread of infectious diseases, the impact of lifestyle factors on health, and disparities in healthcare access. These insights inform policy decisions, such as resource allocation, vaccination campaigns, and public health education programs. Health analysis also aids in evaluating the effectiveness of healthcare interventions and treatments, allowing for continuous improvement in medical practices and healthcare delivery systems. In this way, health analysis not only enhances individual care but also strengthens the overall health infrastructure of communities.
Our Solution
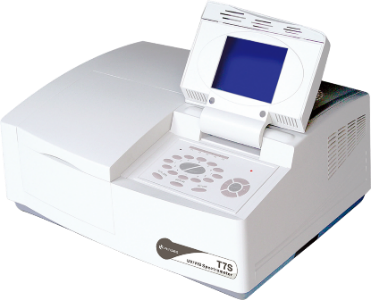
T7S, UV-VIS Spectrophotometer
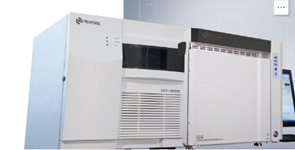
M7 Single Quadrupole GCMS
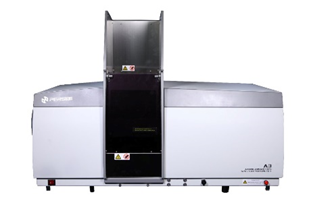
A3AFG-PA: Flame and Graphite Atomic Absorption Spectrophotometer
Processes for Health Analysis
Health analysis involves several key steps aimed at gathering, interpreting, and utilizing health data to assess and improve patient outcomes or public health. These steps ensure a thorough evaluation of individual or population health conditions. Here’s an overview of the different steps in health analysis processes:
- Data Collection
The first step in health analysis is gathering relevant health data. This can include patient medical histories, laboratory test results, vital signs, genetic information, lifestyle factors (e.g., diet, exercise), and environmental influences. Data can be collected through electronic health records (EHRs), surveys, clinical examinations, wearable health devices, or public health databases. Ensuring that the data collected is accurate, comprehensive, and representative is essential for meaningful analysis.
- Data Cleaning and Preparation
Once the data is collected, it must be cleaned and prepared for analysis. This step involves removing errors, duplicate entries, and incomplete records. Data may need to be standardized to ensure consistency across sources, particularly when working with data from multiple healthcare facilities or population studies. Properly cleaning and formatting the data ensures that the subsequent analysis is accurate and reliable.
- Data Classification and Categorization
After cleaning, data is categorized into relevant groups. For example, patient data might be grouped by age, gender, medical condition, or risk factor, depending on the focus of the analysis. Classifying data helps in identifying trends or patterns within specific subgroups. This step is also important for creating control groups in clinical studies or separating different types of health data for analysis (e.g., lab results vs. patient-reported outcomes).
- Statistical Analysis
Statistical methods are applied to analyze the data and draw meaningful conclusions. Techniques such as regression analysis, survival analysis, and hypothesis testing can be used to assess relationships between variables, identify risk factors, and predict outcomes. Statistical analysis helps determine correlations, trends, and causality, which are critical for diagnosing conditions, understanding disease progression, or evaluating the effectiveness of treatments.
- Predictive Modeling
In more advanced health analysis, predictive modeling techniques such as machine learning or artificial intelligence (AI) are used to forecast health outcomes based on existing data. For example, predictive models can estimate the likelihood of developing a certain condition, such as diabetes or heart disease, based on a combination of risk factors. These models can guide preventive measures and personalized healthcare strategies by predicting disease onset or patient responses to treatments.
- Interpretation of Results
Once the data has been analyzed, the results need to be interpreted in the context of the health issue being studied. This involves understanding what the statistical relationships mean for patient care or public health. For example, if the analysis reveals a strong correlation between smoking and lung disease, healthcare providers can emphasize smoking cessation programs. In clinical settings, this step also helps doctors make decisions about diagnosis, treatment options, and patient management.
- Reporting and Documentation
The results of the analysis are compiled into reports that summarize the findings and their implications. These reports may be shared with healthcare providers, public health officials, or researchers, depending on the scope of the study. Documentation may include detailed explanations of the methodology used, the results of statistical tests, and recommendations for clinical or policy action. Reporting is crucial for ensuring transparency and guiding evidence-based healthcare decisions.
- Decision-Making and Implementation
Based on the analysis, healthcare providers, policymakers, or researchers make informed decisions. In clinical practice, this could involve adjusting a patient’s treatment plan, recommending lifestyle changes, or scheduling further tests. On a broader scale, health analysis informs public health interventions, such as vaccination campaigns, resource allocation, or disease prevention initiatives. Implementation of the findings ensures that the analysis translates into practical, actionable outcomes.
- Monitoring and Feedback
After implementing changes based on health analysis, continuous monitoring and feedback loops are essential to evaluate the impact of these decisions. For example, in a public health setting, tracking changes in disease rates after introducing a new health policy helps measure its effectiveness. In clinical settings, monitoring patient outcomes helps assess whether a treatment plan is working as expected, enabling ongoing adjustments to improve patient care.
Each of these steps is critical in turning raw health data into actionable insights that improve patient outcomes, enhance public health strategies, and contribute to better healthcare decision-making.
Analytical Challenges
Health analysis research faces several significant challenges due to the complexity of healthcare data, ethical concerns, and the ever-evolving nature of medical science. Here are some of the key challenges researchers encounter:
- Data Privacy and Security One of the primary challenges in health analysis research is ensuring the privacy and security of sensitive patient data. Health data contains personal information that must be protected in accordance with laws like HIPAA (Health Insurance Portability and Accountability Act) in the U.S. or GDPR in Europe. Ensuring confidentiality while sharing data for research purposes is challenging, as breaches or misuse of data can lead to serious ethical and legal consequences. Anonymizing data is crucial, but even this can be difficult when dealing with complex datasets that may still allow for patient re-identification.
- Data Quality and Inconsistencies
Health data is often collected from multiple sources—hospitals, clinics, labs, wearable devices—and may vary in format, accuracy, and completeness. Incomplete, inaccurate, or inconsistent data can lead to unreliable conclusions. For example, medical records may have missing entries, incorrect diagnoses, or inconsistent coding, which makes standardizing and cleaning the data a time-consuming and difficult process. Poor data quality compromises the validity of the research and makes it harder to derive meaningful insights.
- Heterogeneous Data Types
Health analysis involves different types of data, such as structured data (lab results, diagnoses), unstructured data (doctor’s notes, images), and semi-structured data (EHRs). Integrating and analyzing such heterogeneous data types poses significant technical challenges. For instance, imaging data from MRIs requires different analytical approaches compared to genetic data or patient-reported outcomes. Combining these different data types for comprehensive analysis requires advanced tools and methodologies, which may not be readily available or easy to implement.
- Ethical Concerns and Consent
Health research often involves sensitive patient data, and obtaining informed consent is a major ethical challenge. Patients may not fully understand how their data will be used, especially in long-term or secondary studies. Additionally, in some cases, obtaining consent from large populations or retrospective datasets is difficult, leading to ethical dilemmas regarding data usage. Researchers must balance the need for data to advance health research with respecting patient autonomy and rights.
- Bias and Generalizability
Bias in health data, whether from sampling methods, researcher bias, or healthcare disparities, is a significant challenge. For example, data collected from urban hospitals may not represent rural populations, and certain demographics, such as minority groups or people with lower socio-economic status, may be underrepresented in the datasets. This lack of diversity can limit the generalizability of the findings, making it difficult to apply the results of health analysis research to broader populations.
- Technological and Analytical Complexity
Health data often requires advanced analytical techniques, including machine learning, predictive modeling, and statistical analysis. However, implementing these methods requires specialized skills and technological infrastructure. Not all researchers or healthcare institutions have access to the necessary tools or expertise, which can limit the ability to perform complex analyses. Furthermore, interpreting the results from advanced algorithms can be challenging, especially when results are not easily explainable to non-specialists, such as healthcare providers or patients.
- Regulatory Challenges
Health research is subject to strict regulatory frameworks that govern how data can be collected, used, and shared. These regulations vary by country and can change over time, creating additional complexity for international collaborations or longitudinal studies. Complying with regulations such as HIPAA, GDPR, and others can be resource-intensive, requiring careful data handling, documentation, and sometimes limiting access to valuable datasets for analysis.
- Timeliness and Real-Time Analysis
The dynamic nature of health conditions and public health emergencies, such as disease outbreaks, requires timely analysis. However, delays in data collection, processing, or reporting can hinder the ability to respond effectively. Real-time health data analysis, especially during events like pandemics, is critical, but the infrastructure to collect and analyze such data quickly is often lacking. The delay between data collection and actionable insights can negatively impact patient outcomes or public health measures.
- Cost and Resource Limitations
Health analysis research, especially when large datasets or advanced technologies are involved, can be expensive. The costs of data collection, cleaning, storage, and advanced analytical tools are high, limiting the scope of research, especially for smaller institutions or in low-resource settings. Funding for health research may also be inconsistent or competitive, making it difficult to sustain long-term studies or invest in the necessary infrastructure.
- Evolving Health Landscape
The health landscape is continually evolving due to emerging diseases, advancements in medical technology, and changing patient demographics. Keeping health analysis methodologies up to date with the latest medical knowledge, technologies (e.g., genomics, AI), and societal changes (e.g., aging populations) is a significant challenge. As new treatment protocols or diagnostic tools are introduced, historical data may become outdated, requiring adjustments in analysis techniques or re-interpretation of past findings.
These challenges highlight the complexity of health analysis research and the need for innovative solutions, ethical practices, and cross-disciplinary collaboration to address them effectively.
Features of our T7S UV-VIS, A3AFG UV-VIS and M7 Single Quadrupole GCMS
T7S, UV-VIS (Molecular) | M7 – GCMS | A3AFG (Atomic) |
· Easy to use
| · Easy to use · The new EI source – high ionization efficiency. · The new generation molecular pump · Front Panel: observation window on the front panel · Ion Source: Electron impact source, with dual heating at Max temperature is 350℃. · Dual filament design provides double filament life. · Vacuum System: High performance pre-vacuum pump and turbo molecular pump, to reduce ion collisions and noise. · Quadrupole Mass Analyzer: High precision: metal molybdenum quadrupole mass analyzer, · Removable pre-quadrupole filter device · Detector · Small-signal Amplifier with composite I/V conversion circuit technology, to effectively reduce circuit noise, | · Easy to use · AA 3.0 Win software is a powerful and intuitive software product designed to allow control and data acquisition · Light source: Automatic 8 Hollow Cathode lamp turret controlled and optimized by the AAWin software · Background correction: D2 lamp background correction system and Self-Reversal background correction system fitted as standard to all configurations · Optical system: High precision minimal optics ensure maximum light output · Autosampler: conveniently mounted on the front of the A3 instrument · Flame mode: offers three flame options · Air/Acetylene: High sensitivity (Cu 2ppm >0.280abs) · N2O/Acetylene: less prone to ionization such as: Aluminum, Tin, Titanium, Calcium, Vanadium and Molybdenum · Air/Propane (LPG): is ideal for analyzing alkali metals such as: Potassium, Sodium and Lithium, especially when used in the emission mode · Graphite furnace temperature control Graphite tube: Pyrolytically coated graphite tubes are used as standard |
Read more | Read more | Read more |